Keynotes
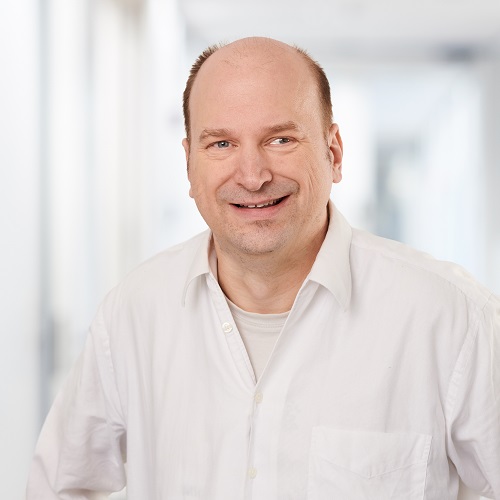
Prof. Dr. Tim Beißbarth
Universitätsmedizin Göttingen
Leveraging biological networks and explainable machine learning for precision oncology.
Precision oncology aims to provide personalized treatment recommendations based on the molecular profile of each patient?s tumor. However, this task is challenging due to the high dimensionality and complexity of genomic data, as well as the limited availability of clinical evidence for rare or novel variants. Therefore, there is a need for computational methods that can integrate prior biological knowledge, such as gene-gene interactions or pathways, and provide interpretable predictions that can support clinical decision making. In this talk, I will present some recent works from the Department of Medical Bioinformatics in Göttingen.
First, I will introduce a method using graph convolutional neural networks (GCNNs) and layer-wise relevance propagation (LRP) to analyze genomic data in the context of biological networks. GCNNs are a type of deep learning model that can learn from graph-structured data, such as molecular networks, and capture the dependencies among features. LRP is a technique that can explain the predictions of GCNNs by assigning relevance scores to each feature, indicating how much it contributes to the output. I will show how these methods can be applied to identify patient-specific molecular subnetworks responsible for metastasis prediction in breast cancer1, and how to perform stable feature selection across different datasets2.
In addition, I will also discuss the challenges and opportunities of implementing precision oncology in clinical practice, focusing on the role of molecular tumor boards (MTBs). MTBs are multidisciplinary teams that review the molecular findings and treatment options for individual patients. I will present tools developed in my department to facilitate the evidence-driven reporting of actionable variants in MTBs3,4. I will demonstrate how these tools can help to streamline the workflow of MTBs and improve the quality and consistency of their reports.
References:
- Chereda H, Bleckmann A, Menck K, Perera-Bel J, Stegmaier P, Auer F, Kramer F, Leha A, Beißbarth T. Explaining decisions of graph convolutional neural networks: patient-specific molecular subnetworks responsible for metastasis prediction in breast cancer. Genome Med, 2021, 13(1):42.
- Chereda H, Leha A, Beißbarth T. Stability of feature selection utilizing graph convolutional neural network and layer-wise relevance propagation. BioRxiv, 2021.
- Perera-Bel J, Hutter B, Heining C, Bleckmann A, Fröhlich M, Fröhling S, Glimm H, Brors B, Beißbarth T. From somatic variants towards precision oncology: Evidence-driven reporting of treatment options in molecular tumor boards. Genome Med, 2018, 10(1):18.
- Kurz NS, Perera-Bel J, Höltermann C, Tucholski T, Yang J, Beißbarth T, Dönitz J. Identifying Actionable Variants in Cancer – The Dual Web and Batch Processing Tool MTB-Report. Stud Health Technol Inform, 2022, 296:73-80.
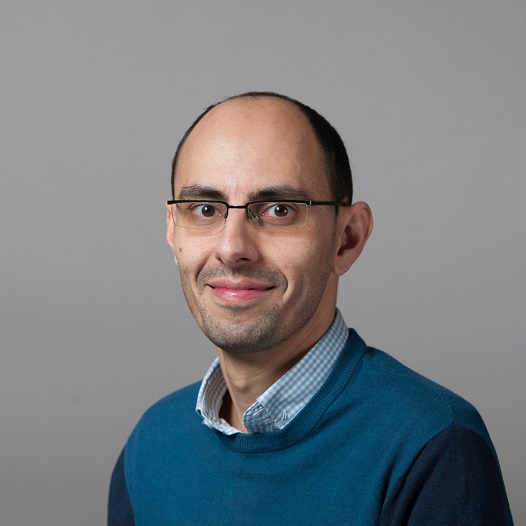
Keywan Hassani-Pak PhD
Head of Bioinformatics at Rothamsted Research
Unveiling the Narratives of Complex Traits and Diseases: Integrative Bioinformatics enhanced with AI.
In this captivating keynote presentation, we embark on a transformative journey into the world of integrative bioinformatics, where we explore the profound impact of knowledge integration in unraveling the narratives behind complex traits and diseases. Through advanced computational techniques, we discover how the seamless fusion of diverse data sources creates a cohesive knowledge graph that transcends traditional data silos. We will delve into the challenges and opportunities of integrative bioinformatics, where genomics, genetics, and literature data converge to form a comprehensive knowledge landscape. Witness how this integration empowers researchers to extract meaningful insights, generate hypotheses, and gain a deeper understanding of the intricate web of biological relationships.
Throughout the presentation, we showcase the transformative impact of KnetMiner, a powerful knowledge platform used in gene and trait discovery. Its cutting-edge capabilities accelerate knowledge mining, advance hypothesis generation, and foster improved research outcomes. By telling the stories of complex traits and diseases, KnetMiner has reshaped our understanding of biology and provided us with the essential tools to unravel the mysteries of many plant and animal genomes. We conclude with an outlook into the future of knowledge discovery, embracing the profound impact of AI, including Large Language Models, on our scientific quest. We explore the fusion of knowledge graphs and language models, unveiling the advancements achieved through their harmonious integration. Together, they pave the way for innovative discoveries and a deeper comprehension of the ever-evolving biological world.
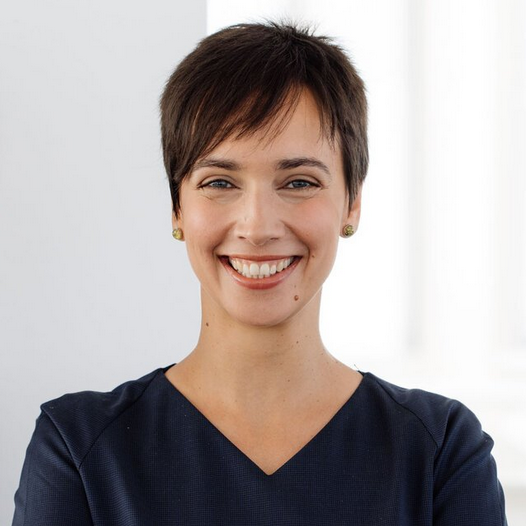
Jun.-Prof. Dr. Anna Matuszyńska
RWTH Aachen University Germany
Computational Models of Photosynthesis: Leveraging Single Time Course to Large-Scale, Heterogeneous Datasets.
Photosynthetic organisms perform highly efficient energy converting reactions despite constant exposure to ever-changing environmental conditions. If not to changes in the temperature, then to water availability, or light; or are even attacked by pathogens or herbivores. To dynamically adapt to these changes, numerous processes have evolved and been acquired, of which some affect plants’ photosynthetic performance. In our Lab we are using computational models to systematically increase our quantitative understanding of how photosynthesis is regulated and to provide our experimental partners with new targets for improved biomass/metabolite production.
In this talk I will first briefly present our recent advances in utilizing computational methods to capture the dynamic behavior of photosynthesis in cyanobacteria and plants. Next, I will focus on the work investigating the role of photosynthesis inside of plants epidermal outgrowths named glandular trichomes. Through the integration and alignment of diverse datasets, including multi-omics data, metabolic pathway databases (LycoCyc and MetaCyc), and the COBRA Toolbox, we have developed a stoichiometric model of specialized metabolism in photosynthetic glandular trichomes of Solanum lycopersicum (tomato). Our computational model not only predicts the shift in carbon partitioning under varying light intensities but also sheds light on the role of primary metabolism in driving high metabolic fluxes within glandular trichomes. By incorporating integrative bioinformatics approaches into the study of metabolism in photosynthetic organisms, we predicted that increasing light intensities results in a shift of carbon partitioning from catabolic to anabolic reactions driven by the energy availability of the cell. Moreover, we show that the photosynthetic activity of the cell provides an added benefit of shifting between two isoprenoid pathways under different light regimes.
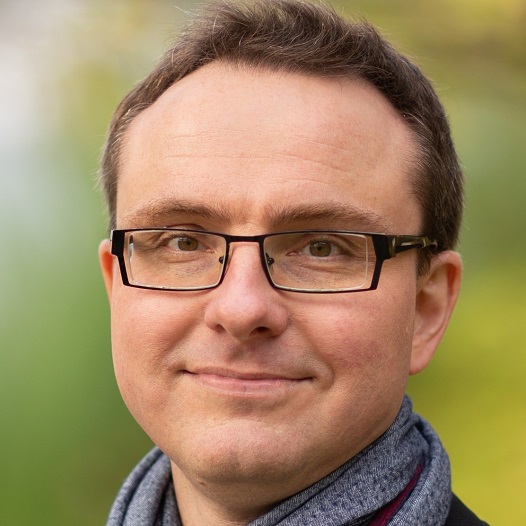
Prof. Janusz Bujnicki
International Institute of Molecular and Cell Biology Warsaw
Computational modeling of RNA 3D structures and interactions – with and without the use of experimental data.
Ribonucleic acid (RNA) molecules are master regulators of cells. They play key roles in many molecular processes: transmitting genetic information, sensing cellular signals, relaying responses, and even catalyzing chemical reactions. The function of RNA, especially its ability to interact with other molecules, is encoded in its sequence. To understand how these molecules carry out their biological tasks, we need detailed knowledge of RNA structure, dynamics, and thermodynamics. The latter largely determines how RNA folds and interacts within the cellular environment.
Experimentally determining these properties is challenging. Several computational methods have been developed to model the folding of RNA 3D structures and their interactions, mainly with proteins. However, these computational methods are nearing their limits, especially when the biological implications demand calculations of dynamics beyond a few hundred nanoseconds. For researchers facing such challenges, a more effective approach is to use coarse-grained modeling. This reduces both the data points and computational effort to a feasible level, while retaining as much essential information as possible.
I will present strategies for computational modeling of RNA 3D structures and their interactions with other molecules. These strategies use a suite of methods from my laboratory, based on the SimRNA program. Our methods employ coarse-grained representations of molecules, utilize the Monte Carlo method for sampling conformational space, and use statistical potentials to approximate energy. They also help identify conformations that match biologically relevant structures. Specifically, I will discuss computational methods to determine RNA structure using low-resolution experimental data, such as chemical probing and electron microscopy.
References:
- Ponce-Salvatierra, A. et al. Biosci. Rep. 39, BSR20180430 (2019)
- Boniecki, M. J. et al. Nucleic Acids Res. 44, e63 (2016)
Bujnicki is a Professor of Biology and leads a research group at IIMCB. His group integrates bioinformatics with structural biology, focusing on understanding how RNA molecule sequences encode their 3D structures, and determine interactions and biological functions. Bujnicki’s research interests encompass the combination of theory with experiment, integration of various data types, molecular simulations, scientific uncertainties, and the interplay between science and decision-making. His notable contributions to science include the development of methods for computational modeling of protein and RNA 3D structures, as well as the discovery and characterization of enzymes involved in post-transcriptional modification of RNA. He has authored over 350 publications, which have been cited more than 13,000 times.
Currently, the Bujnicki group focuses on experimental determination and theoretical analyses of RNA structures and their complexes with other molecules, using computational modeling together with cryo-electron microscopy, X-ray crystallography, and other biochemical and biophysical techniques. One of the goals of their research is to identify small chemical molecules that act on RNAs involved in various diseases (e.g., viral genomic RNAs) and could be candidates for future drugs.
Bujnicki has received multiple awards for his scientific achievements. He has been elected as a member of the Polish Academy of Sciences, European Molecular Biology Organization (EMBO), and Academia Europaea. He is an alumnus of the Leadership Academy for Poland.
Beyond his research, Bujnicki is active as a science advisor. He has served as a member of the European Commission’s Group of Chief Scientific Advisors (GCSA) and continues to collaborate with the GCSA as an expert. He has also been involved in several science-for-policy advisory bodies for the Polish Ministry of Science and held the position of Chairman of the University Council at the University of Warsaw. For his significant contributions at the intersection of science and policy-making, Bujnicki was awarded the André Mischke Prize by the Young Academy of Europe.